Drug development: increasing efficiency with specific platforms?
Bildnachweis: CHONCHANOK PHOTO – stock.adobe.com, PitchBook Data, Inc. All rights reserved., Quelle: Copyright 2024 PitchBook Data, Inc. All rights reserved..
From the perspective of venture capital (VC) investors, AI applications that take drug development to a new level are very attractive. They promise great time and cost savings. But how far are startups working with powerful AI platforms? And what specific questions can be used to add value?
The traditional way of drug development is time-consuming, risky and very expensive. It takes about ten to 15 years and usually costs upwards of USD 2 billion from discovery to the launch of a drug on the market. High R&D costs, coupled with dismal drug failure rates, place a huge financial burden on the biotechnology and pharmaceutical industries. good and social. The combination of AI and biology promises to help. AI is seen as the future game changer in drug development.
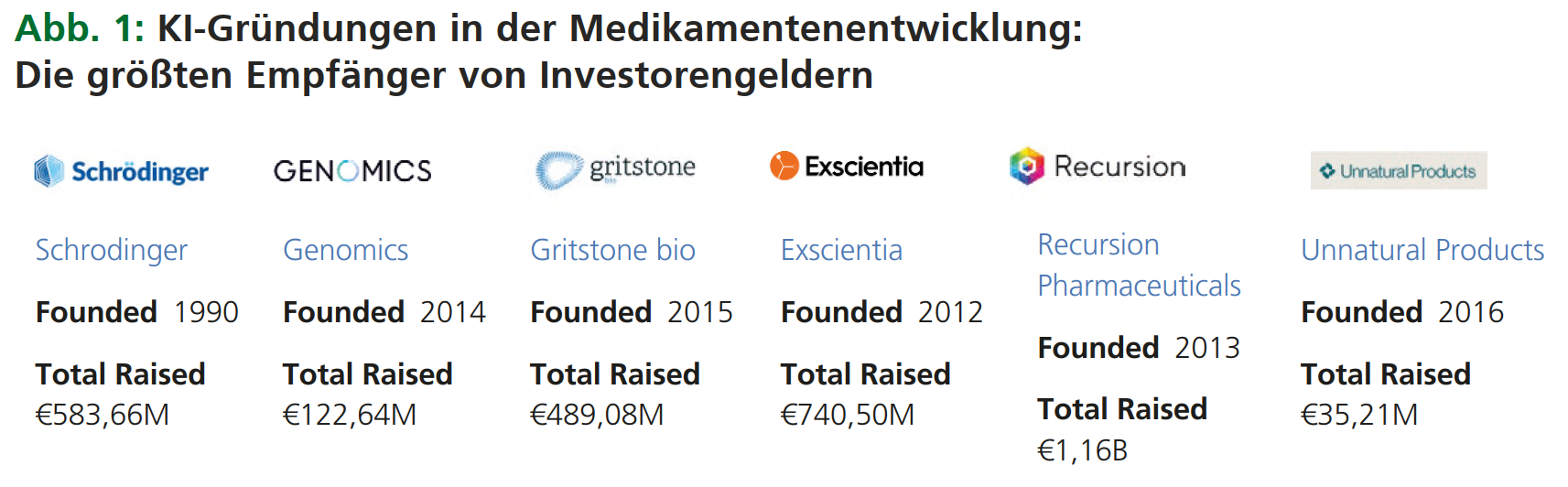
The requirements for this are there: For example, with AI, researchers can discover new biological molecules and use multiomics to analyze biological data at lightning speed. You can accurately and quickly predict how drugs will interact with targets and identify possible side effects. All this significantly shortens, improves and reduces the development time of new drugs.
But where do start-ups stand when it comes to using AI?
There is currently no drug for which AI has played a significant role in the stone development process leading to market approval. However, many startups have been working in the market for many years to achieve this goal. PitchBook lists 186 well-known companies using AI applications in drug development. About 1,200 investors have so far invested more than EUR 16 billion in these companies. There have been 854 deals in the industry since 2018. Over the past twelve months, the number of deals has dropped by 13% to 64, but the amount invested has increased by 24% to about EUR 2.5 billion. Creation of AI-focused start-ups
Although drug development had been increasing until the mid-2010s, the number of new startups has recently decreased, according to PitchBook. The highest investor funds went to Recursion Pharmaceuticals (EUR 1.16 billion), Exscientia (EUR 740 million), Schrodinger (EUR 583 million) and Gritstone bio (EUR 489 million).
These figures show the dimensions that the sector described here has achieved so far. Looking at the most important funding categories and deals, it is also clear that the majority of start-ups in this sector are based in the USA, supplemented by a small group in London/UK. What can be concluded from this?
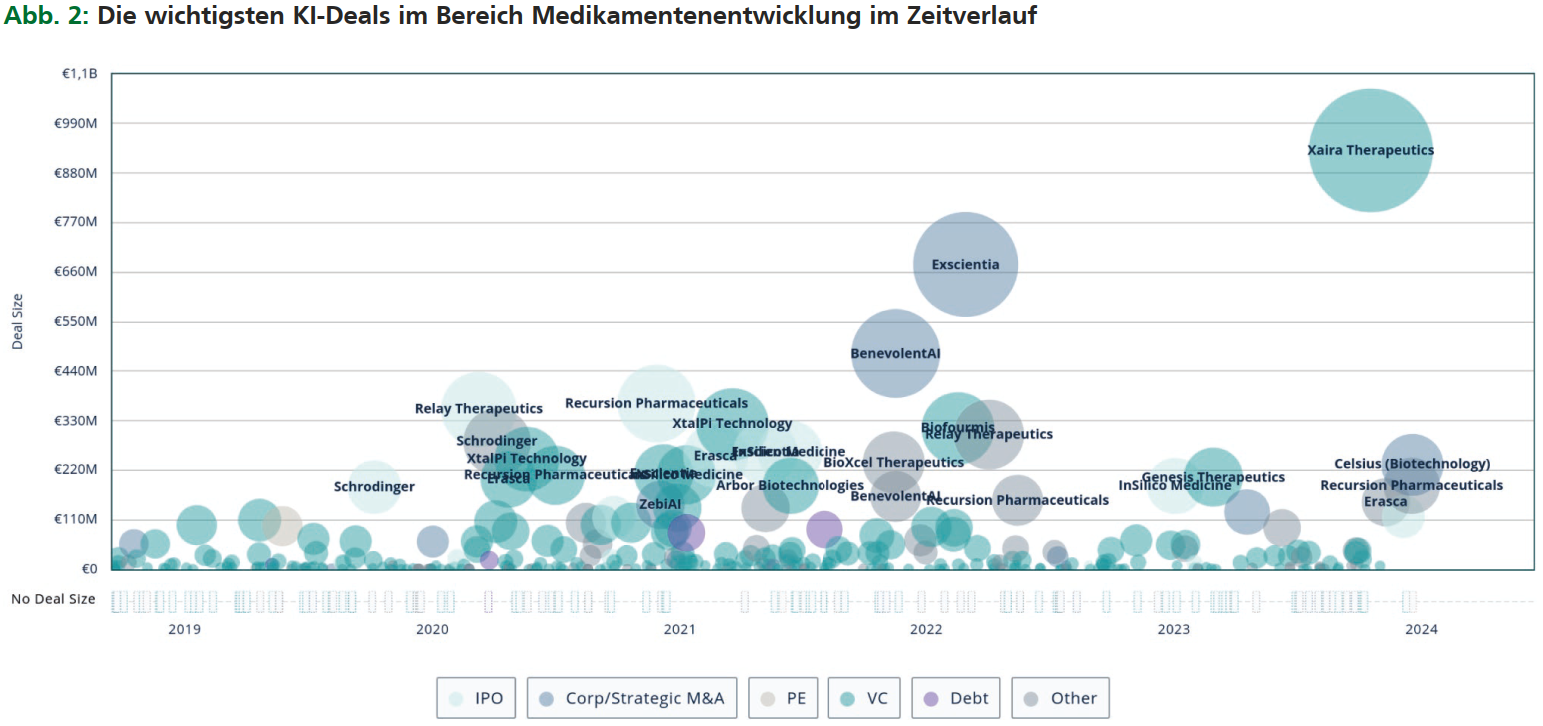
Where AI platforms can be used in particular
When it comes to the use of software and AI, drug development must first answer an important question: In what areas can AI contribute to time and cost efficiency? We see the following processes here. Accelerating target identification and validation: This early and critical phase of drug development previously required laborious laboratory work and a lot of trial and error. AI platforms, especially those targeted at specific diseases, can facilitate this process by analyzing large amounts of data and filtering potential targets. These platforms can predict which proteins or genes are most likely to be associated with disease. Researchers then focus their efforts on the most promising candidates.
- Enhanced Screening and Lead Development: Once potential targets are identified, the next step is to screen thousands of chemicals to find those that interact with the target in the desired way. AI platforms in pathology can simulate these interactions on a computer, greatly reducing the need for physical examination. These platforms can also improve formulations by predicting how changes in chemical composition will affect their efficacy and safety.
- Personalized medicine and patient stratification: Disease-specific AI platforms are also playing an important role in personalized medicine. By analyzing patient data, these platforms can identify subgroups of patients who are most likely to respond to a particular treatment. This allows the development of medicines that are adapted to a certain number of patients, increasing their effectiveness and reducing the possibility of side effects. In addition, personalized medicine reduces the cost of clinical trials by ensuring that the right patients are selected.
- Reducing the cost and time of clinical studies: Clinical studies drive the cost of drug development. Disease-related AI platforms significantly reduce these costs by improving patient recruitment and triage, predicting outcomes, and monitoring patient responses in real time. By identifying the most qualified candidates for testing, they increase their efficiency and shorten their time. They also reduce the chances of failure.
- Integration of real-time data and market monitoring: Once a drug is approved and brought to market, it is important to monitor its performance in the real world. Disease-related AI platforms can integrate real-time data, such as electronic health records and patient-reported outcomes. This ongoing monitoring helps identify long-term problems and benefits that may not have been apparent during clinical trials. Furthermore, by analyzing these data, AI platforms can contribute to the development of new treatments and the improvement of existing medicines.
A bright future is in sight
Disease-specific AI platforms are on the way to revolutionizing drug development. We expect strong development and use of drug development. Three important factors, each of which brings about the necessary growth, contribute to this: Continuously improving AI and large-scale linguistic models combine with many tools based on omics technology – and these are explained by increasing computing power for data analysis.
#Drug #development #increasing #efficiency #specific #platforms